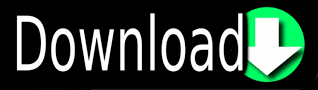
#ACCURATE 4 FULL FREE#
(28) Recently, alchemical free energy calculations have also shown promise for the prediction of protein thermostability and drug resistance. (4,22,25) Free energy calculations based on first-principles statistical mechanics that make use of nonphysical (i.e., alchemical) pathways in a thermodynamic cycle have become increasingly popular in small molecule drug discovery for the optimization of lead compounds (26,27) and have now started being used prospectively by the pharmaceutical industry. (21−24) The limitations of Rosetta have often been ascribed to a limited treatment of backbone flexibility and lack of explicit solvation, so that computational approaches that tackle these challenges may provide a more accurate estimation of ligand affinity changes upon protein mutation. However, our ability to engineer ligand-binding proteins (e.g., biosensors and enzymes) is still limited, and current approaches rely heavily on experimentation, in particular at the optimization stage. (15−18) Other design approaches that have been proposed involve ligand docking to known protein structures (19) or de novo design of short protein sequences using a combination of docking, molecular dynamics (MD), and Monte Carlo simulations. A similar approach has also been employed for the design of artificial enzymes by using a model of the transition state as the target ligand. After experimental optimization of the most promising design, a protein with sub-nanomolar affinity for the steroid was found. Among the 17 computationally designed proteins, two were binding to digoxigenin in the micromolar range. (13) engineered protein binders to the steroid digoxigenin by first designing a minimal ligand-binding shell, then searching the protein data bank (14) (PDB) for suitable scaffolds, and finally optimizing the designs experimentally. (4−10) Fast computational approaches that rely on mixed physics- and knowledge-based potentials, such as Rosetta, (11,12) have already proven successful in the early stages of the discovery process. (1−3) Given their high affinity and selectivity, nontoxicity, and biodegradability, the design of proteins with novel ligand-binding functions also holds great potential for application in biomedicine and biotechnology. Ligand-binding proteins play essential roles in living organisms, with interactions between small organic molecules and proteins triggering a multitude of signal transduction processes. The currently achievable accuracy offers the prospect of being able to employ computation for the optimization of ligand-binding proteins as well as the prediction of drug resistance.
#ACCURATE 4 FULL FULL#
These closely match the experimentally determined ΔΔ G values, with a root-mean-square error of 1.2 kcal/mol for the full benchmark set and of 0.8 kcal/mol for a subset of protein systems providing the most reproducible results. We show that both the free energy calculations and Rosetta are able to quantitatively predict changes in ligand binding affinity upon protein mutations, yet the best predictions are the result of combining the estimates of both methods. After evaluating different protocols with computational efficiency in mind, we investigate the performance of different force fields. Here, we assess the ability of free energy calculations based on first-principles statistical mechanics, as well as the latest Rosetta protocols, to quantitatively predict such affinity changes on a challenging set of 134 mutations. While computational methods have proven successful in the early stages of the discovery process, optimization approaches that can quantitatively predict ligand affinity changes upon protein mutation are still lacking. Computation could reduce the cost of the development process and would allow rigorous testing of our understanding of the principles governing molecular recognition. However, our ability to engineer ligand-binding proteins is still limited, and current approaches rely primarily on experimentation. The design of proteins with novel ligand-binding functions holds great potential for application in biomedicine and biotechnology.
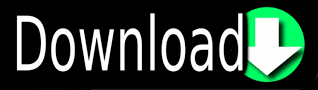